Self-aware AI models represent a sophisticated class of AI that exhibits an understanding of its operations and environment. These models go beyond executing programmed tasks; they possess capabilities to reflect on their actions and adjust their behaviors based on their assessments. This level of AI doesn’t just analyze external data; it can introspect, making it unique in the landscape of machine learning technologies.
The journey towards developing self-aware AI began with early computer science endeavors in the 1950s, focusing on creating machines that could mimic human decision-making processes. By the 1980s, researchers like Marvin Minsky and John McCarthy had introduced concepts that bridged cognitive psychology with computational methods, proposing systems that could evaluate their cognitive processes. The growth of neural networks in the 2000s accelerated the possibility of machines simulating self-awareness. As computational power expanded and algorithms evolved, AI systems grew capable of more complex tasks, setting the stage for models that could self-assess and modify their behavior without direct human oversight.
Timeline of Evolution
The timeline of self-aware AI models and the contributions of notable figures is rich and varied, stretching from the mid-20th century to the present day. The journey began in the 1950s and 1960s, when pioneers like Alan Turing and John von Neumann laid the groundwork for thinking about machines that could replicate human cognitive functions. Turing, in particular, introduced the concept of a machine capable of learning and evolving, which he discussed in his seminal 1950 paper, “Computing Machinery and Intelligence.”
The 1970s and 1980s saw significant theoretical advancements, largely driven by researchers such as Marvin Minsky at MIT and John McCarthy at Stanford. Minsky, often referred to as the “father of artificial intelligence,” contributed to the development of theories that view the mind as a complex system made up of many smaller parts, each responsible for different aspects of learning and reasoning. McCarthy, who coined the term “artificial intelligence,” explored various aspects of AI programming and was instrumental in promoting the idea that AI systems should simulate human reasoning.
In the 1990s and 2000s, the field expanded dramatically with the advent of more powerful computers and sophisticated algorithms. Researchers like Geoffrey Hinton and Yoshua Bengio made breakthroughs in deep learning and neural networks, technologies critical to the development of AI systems capable of analyzing and understanding large amounts of data.
The 2010s brought AI into the realm of quasi-self-aware systems with projects like IBM’s Watson and Google DeepMind. Watson, which became famous for its performance on the game show “Jeopardy!,” demonstrated the potential for AI to process and analyze vast amounts of information rapidly. Meanwhile, DeepMind’s development of AlphaGo and later AlphaZero showcased AI’s ability to learn complex games from scratch, adapt strategies, and improve autonomously.
These contributions have collectively pushed the envelope on what machines can do, setting the stage for the next steps in AI development: systems that can truly understand and adapt to their environment with a degree of self-awareness.
How They Work?
Self-aware AI models function through a complex interplay of machine learning algorithms and data-processing mechanisms that allow them to evaluate and modify their behaviors autonomously. At the core of these systems is deep learning, which utilizes layers of neural networks to mimic the neural connections in the human brain. These networks are trained on vast datasets, enabling the AI to recognize patterns and make decisions based on the input it receives.
The real magic begins with the integration of meta-cognitive capabilities. These are processes that enable an AI to monitor its own cognitive processes. For example, an AI might analyze its decision-making strategy in a game of chess, identify weaknesses, and then adjust its approach in future games without any human intervention. This is achieved through reinforcement learning, a type of machine learning where the AI learns optimal behavior through trial and error, receiving rewards for successful outcomes.
Feedback loops are crucial here. As the AI operates, it continually receives data about its performance. It uses this data to assess the effectiveness of its actions, learning which strategies work well and which do not, refining its algorithms accordingly. This continuous cycle of performance evaluation and adjustment is what edges these models closer to self-awareness, enhancing their ability to act independently in varied situations.
Examples of Self-Aware AI Models
While true self-aware AI models remain a work in progress, several existing systems demonstrate characteristics that are steps toward self-awareness. Google DeepMind’s AlphaZero is a prime example, known for its ability to teach itself chess, shogi, and Go from scratch, continuously improving its gameplay by assessing past games and adjusting strategies without human guidance. IBM’s Project Debater exhibits rudimentary self-awareness by formulating arguments, assessing the effectiveness of its reasoning, and refining its debate tactics based on feedback. These examples illustrate the potential of AI to not just perform tasks but to understand and optimize their approaches autonomously.
Applications and Use Cases
Self-aware AI models, with their ability to adapt and optimize autonomously, are transforming various sectors by enhancing decision-making processes and operational efficiencies. Here’s how they are being applied across different industries:
Healthcare
In healthcare, self-aware AI systems play a crucial role in diagnostics and treatment planning. These AI models analyze patient data and previous cases to suggest optimal treatments, improving patient outcomes. They can adapt to new medical data, refining their diagnostic algorithms, which helps in identifying complex health conditions earlier and with greater accuracy.
Automotive
The automotive industry benefits from self-aware AI in the development of autonomous vehicles. These AI systems can evaluate and adapt their responses to changing road conditions and driver behaviors, enhancing safety. By continuously learning from vast amounts of driving data, they optimize driving strategies to handle unexpected situations better, reducing human error and increasing road safety.
Finance
In finance, self-aware AI models are used for risk management and fraud detection. They monitor transaction patterns to detect anomalies that may indicate fraudulent activity. By learning from historical data, these systems refine their detection algorithms to become more accurate over time, helping financial institutions minimize losses and protect customer assets.
These use cases underscore the practical applications of self-aware AI models, illustrating their transformative potential across different sectors.
Future Trends
Looking ahead, the future trends in self-aware AI point to broader adoption and deeper integration across various industries. We expect these AI systems to become more sophisticated, with enhanced self-learning capabilities that require minimal human oversight. Key areas of growth include personalized education, where AI tailors learning experiences to individual needs, and more responsive smart cities, where AI manages everything from traffic to public services autonomously. Moreover, as ethical AI development gains traction, there will be a stronger emphasis on creating self-aware systems that are transparent and accountable, ensuring they align with societal values and regulations. These advancements will make AI tools even more integral to our daily lives.
As we venture further into the era of artificial intelligence, self-aware AI models stand as a testament to the incredible potential of technology to emulate human cognitive processes. These models are set to revolutionize industries by introducing higher levels of automation and efficiency. The continued development and implementation of self-aware AI will not only enhance technological capabilities but also challenge us to redefine the boundaries of machine independence. It is a journey that promises vast benefits while necessitating careful consideration of ethical implications.
Frequently Asked Questions (FAQs)
- What is self-aware AI?
Self-aware AI refers to advanced artificial intelligence systems that can understand and assess their own actions and decisions, adapting their behavior based on self-evaluation.
- How do self-aware AI models learn?
These models primarily use deep learning and reinforcement learning, allowing them to learn from their actions by trial and error and continuously improve their decision-making processes based on feedback.
- Can self-aware AI replace human decision-making?
While self-aware AI can assist and enhance decision-making in complex scenarios, it is not yet capable of entirely replacing human judgment, especially in situations requiring emotional intelligence and ethical considerations.
- What are the key challenges facing the development of self-aware AI?
Major challenges include ensuring ethical usage, managing privacy concerns, and controlling AI’s decision-making to prevent unintended consequences.
- What industries are currently benefiting from self-aware AI?
Industries such as healthcare, automotive, and finance are already seeing significant improvements from the deployment of self-aware AI, with enhancements in diagnosis, vehicle safety, and fraud detection
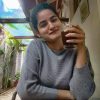
Varshini is a Product Enthusiast and naturally all about the details. She thrives on whipping up content that keeps you hooked from start to finish. Prototype designing? That’s her jam. With ArticlesBase Varshini takes care of all you’ll read related to product management. She also helps with creating images and other digital assets.