Artificial Intelligence (AI) is about creating machines that can perform tasks usually requiring human intelligence. These tasks range from recognizing speech to making decisions. The surge in AI’s popularity is fueled by its vast potential to boost efficiency and solve complex problems across various industries. According to a 2023 report, the AI market is expected to reach $126 billion by 2025, reflecting its critical role in driving innovation. Businesses leverage AI to enhance customer experiences, streamline operations, and create new products. Moreover, advancements in computing power and data availability have propelled AI development, making it more accessible and effective across sectors. This growing integration is why understanding AI is becoming essential for everyone.
Why it is important to learn the basics of AI
Learning the basics of AI is crucial because it influences a broad range of technologies that permeate our daily lives. From personalized shopping recommendations to automated customer support, AI enhances user experiences and operational efficiency across multiple sectors. Understanding AI helps individuals make informed decisions about using technology, discerning between beneficial applications and potential risks such as privacy concerns. For professionals, knowledge of AI opens up opportunities for career advancement and innovation in almost any field, not just technology.
As AI continues to evolve, being familiar with its fundamental concepts will be essential for adapting to new developments and participating effectively in discussions and decisions related to AI applications in both professional and personal contexts.
List 30 AI terms and terminologies
Before diving into specific applications and technologies, it’s essential to understand some foundational terms in AI. Here’s an expanded look at three key terms that form the bedrock of artificial intelligence discussions:
- Algorithm – An algorithm is a sequence of instructions designed to perform a specific task. In AI, algorithms process data and make decisions, mimicking the logical reasoning humans might use to solve the same problem. They can range from simple formulas to complex decision trees.
- Artificial Intelligence (AI) – Artificial Intelligence refers to the capability of a machine to imitate intelligent human behavior. By integrating algorithms, machines perform tasks such as learning, reasoning, and self-correction. This broad field encompasses other areas like machine learning and robotics, making it a pivotal innovation driver.
- Machine Learning (ML) – Machine Learning is a subset of AI where machines learn from data without explicit programming. Using statistical techniques, ML models identify patterns and make decisions. This learning process improves with more data, enhancing the machine’s ability to perform tasks over time effectively.
- Deep Learning – Deep Learning is an advanced subset of machine learning that uses multi-layered neural networks to analyze various data types. These networks mimic the human brain’s structure and function, allowing machines to recognize patterns and make decisions with minimal human intervention. Deep learning excels in tasks such as image and speech recognition.
- Neural Network – A neural network is a series of algorithms that endeavors to recognize underlying relationships in a set of data through a process that mimics the way the human brain operates. These networks can adapt to changing input, ensuring the generation of the best possible result without needing to redesign the output criteria.
- Natural Language Processing (NLP) – Natural Language Processing is a technology that allows computers to understand, interpret, and respond to human languages in a way that is both valuable and meaningful. NLP combines computational linguistics with machine learning to process and analyze large amounts of natural language data.
- Computer Vision – Computer Vision is a field of AI that trains computers to interpret and understand the visual world. Using digital images from cameras and videos and deep learning models, machines can accurately identify and classify objects, and then react to what they “see” just as humans do.
- Chatbot – A chatbot is a software application used to conduct an online chat conversation via text or text-to-speech, in lieu of providing direct contact with a live human agent. Designed to convincingly simulate how a human would behave as a conversational partner, chatbots are typically used in customer service for both information acquisition and customer assistance.
- Supervised Learning – Supervised learning is a type of machine learning where models are trained using labeled data. The system learns to predict outcomes by being taught what the correct answer or outcome should be for each data input. This method is widely used for applications where historical data predicts likely future events.
- Unsupervised Learning – Unlike supervised learning, unsupervised learning involves training an AI model on data without pre-labeled answers. The model tries to identify patterns and relationships directly from the data itself, commonly used for clustering and association tasks where the structure of data is unknown.
- Reinforcement Learning – Reinforcement learning is a type of machine learning where an AI agent learns to make decisions by performing certain actions and experiencing the results. The agent learns from past actions to learn better through trial and error, making it effective for real-time decisions in complex environments.
- Classification – Classification in AI refers to the process of predicting the category to which a new observation belongs. AI systems are trained with data that is already categorized to recognize where new data will fit, such as sorting emails into spam and non-spam categories.
- Regression – Regression is a statistical method used in AI to predict the relationship between variables and forecast continuous values. It helps in understanding how the value of the dependent variable changes when any one of the independent variables is varied, typically used for predicting prices, temperatures, or any quantitative output.
- Decision Tree – A decision tree is a model that uses a tree-like graph of decisions and their possible consequences. It’s like following a path of yes/no questions until you reach the end. This method is great for making clear and straightforward decisions based on various inputs.
- Random Forest – Think of a random forest as a team of decision trees working together. Each tree in the random forest makes its own decision, and then they vote to decide on the final output. This method increases accuracy by correcting the mistakes of individual trees.
- Gradient Boosting – Gradient boosting is a way to refine predictions in AI. It works by sequentially correcting errors made by previous predictions with new models. This approach gradually improves the accuracy of the predictions, making the model smarter with each step.
- Clustering – Clustering involves grouping a set of objects so that objects in the same group are more similar to each other than to those in other groups. It’s used widely to find natural groupings among data, such as grouping customers by purchasing behavior.
- Dimensionality Reduction – Dimensionality reduction simplifies data by reducing the number of variables under consideration, using methods that preserve the essence of the data. This is especially useful when dealing with huge datasets, as it helps to focus on the most important information.
- Feature Engineering – Feature engineering involves selecting, modifying, or creating new features from raw data to improve the performance of machine learning models. It’s like fine-tuning inputs to help models learn better and make more accurate predictions.
- Generative Adversarial Network (GAN) – A GAN consists of two neural networks contesting with each other. One generates candidates while the other evaluates them. This setup helps in refining outputs, as the generator strives to make indistinguishable imitations, improving through the critic’s feedback.
- Convolutional Neural Network (CNN) – CNNs are specialized in processing data that has a grid-like topology, such as images. These networks use filters to capture spatial hierarchies in data by recognizing patterns and features like edges, shapes, and textures, making them powerful for image and video analysis.
- Recurrent Neural Network (RNN) – RNNs are designed to handle sequential data, like text or time series. They can remember previous inputs in the sequence, using this memory to influence the output, which makes them ideal for tasks where context is crucial, such as language translation or speech recognition.
- Anomaly Detection – Anomaly detection identifies unusual patterns that do not conform to expected behavior. It is crucial in fraud detection, network security, and fault detection, providing alerts whenever something out of the ordinary occurs, helping prevent potential issues before they escalate.
- Natural Language Generation (NLG) – NLG transforms structured data into natural language. It enables computers to write reports, generate text-based content, and even author entire articles, making it invaluable for automating content creation and enhancing user interactions with tech devices.
- Robotics – Robotics combines engineering and AI to create robots that perform tasks autonomously. These machines can operate in environments too hazardous for humans, perform precise operations in medical surgery, or handle repetitive tasks in manufacturing, significantly increasing efficiency and safety.
- Data Mining – Data mining involves extracting valuable information from large datasets. It uses statistical methods to discover patterns and relationships that can inform decision making. This process is fundamental in sectors like marketing, where understanding consumer behavior can drive sales strategies.
- Sentiment Analysis – Sentiment analysis uses NLP to determine the emotional tone behind a series of words. This is useful for businesses to understand customer opinions on social media, enabling them to tailor services or products to better meet consumer needs.
- Predictive Analytics – Predictive analytics uses historical data and statistical algorithms to forecast future events. Widely used in fields ranging from marketing to weather forecasting, it helps organizations make informed decisions by predicting trends and consumer behaviors.
- Cognitive Computing – Cognitive computing aims to replicate human thought processes in a computerized model. It involves self-learning systems that use data mining, pattern recognition, and natural language processing to mimic the way the human brain works. This technology is often used to solve complex problems where answers may be ambiguous and unclear, making it invaluable in areas like customer support and healthcare diagnostics.
- Bias in AI – Bias in AI refers to an AI system’s tendency to make unfair or prejudiced decisions due to flawed assumptions in the algorithm or biases in the training data. It can manifest in various ways, such as racial bias in facial recognition software or gender bias in job recommendation systems. Addressing AI bias is crucial to developing fair and ethical AI systems that make decisions beneficially and justly for all users.
In conclusion, understanding these 30 AI terms provides a solid foundation for anyone interested in the rapidly evolving field of artificial intelligence. Whether you’re a student, a professional, or just curious about AI, knowing these terms will help you grasp the essential concepts and engage more deeply with the technology shaping our world.
FAQs
- What is AI and how is it used?
Artificial Intelligence (AI) simulates human intelligence in machines to perform tasks like reasoning, learning, and problem-solving. It’s used in various industries for tasks such as automating processes, enhancing customer service, and improving decision-making.
- Why is machine learning important in AI?
Machine learning is crucial because it allows computers to learn and adapt from experience without being explicitly programmed. This capability is at the core of making AI systems more efficient and effective over time.
- What are the differences between AI and robotics?
AI involves creating software that can think intelligently, whereas robotics deals with the physical creation of robots. AI can be a component of robotics when robots use AI to perform tasks autonomously.
- How does natural language processing benefit businesses?
Natural Language Processing (NLP) allows businesses to analyze and understand human language, enabling better customer interaction, sentiment analysis, and automated support, thus enhancing overall customer satisfaction.
- What is the significance of data mining in AI?
Data mining is significant in AI as it involves extracting patterns from large data sets to learn and make informed decisions. This process is fundamental for predictive analytics, helping businesses foresee trends and behaviors.
- How can we address bias in AI systems?
Addressing bias in AI involves using diverse and inclusive data sets during training, continually testing and refining AI models, and implementing ethical guidelines to ensure AI systems perform fairly and equitably.
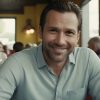
Chris White brings over a decade of writing experience to ArticlesBase. With a versatile writing style, Chris covers topics ranging from tech to business and finance. He holds a Master’s in Global Media Studies and ensures all content is meticulously fact-checked. Chris also assists the managing editor to uphold our content standards.
Educational Background: MA in Global Media Studies
Chris@articlesbase.com